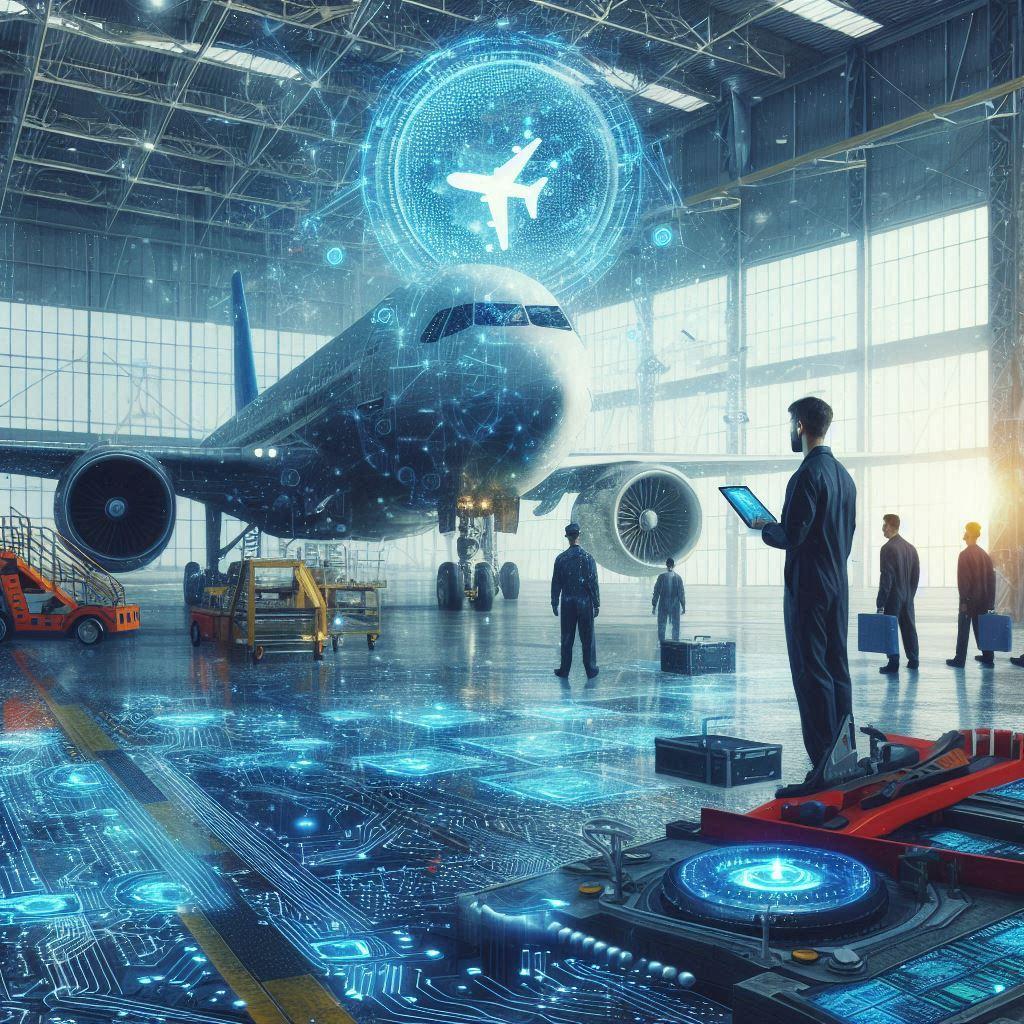
While aircraft maintenance, repair and overhaul (MRO) may seem at first glance like a predominantly physical undertaking, it’s actually an area that stands to be transformed by AI and machine learning. Jon Burnip, Chief Technology Officer – Aerospace at Marshall, explains how this could happen.
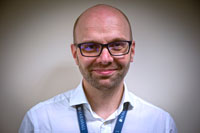
In 20 years since joining Marshall, Jon Burnip has progressed from a university placement to the role of Aerospace Chief Technology Officer (CTO). Along the way, he has held a range of roles ranging from design engineering and stress analysis to durability testing and systems engineering.
Jon is now responsible for developing and implementing Marshall’s Aerospace technology roadmap, focusing on future product opportunities and new approaches to delivering extraordinary products for our customers.
Very briefly, what would you say are the biggest the opportunities for AI in aircraft maintenance, repair and overhaul (MRO)?
From my perspective, there are three key areas where MRO providers could apply AI to unlock value for aircraft fleet operators.
The first is predictive maintenance, where AI can help predict damage or failures before they become serious or cause delay, saving cost and minimising aircraft downtime.
Next, there are opportunities for task optimisation, which would involve using maintenance data to generate insights into how MRO work can be planned and executed more efficiently.
The third key area, in my view, is augmentation of human practice, where AI can enhance the capabilities of MRO technicians, offer insights and guide decision-making.
What is the problem that predictive maintenance could solve?
In the course of heavy maintenance we frequently discover large amounts of “emergent work” - we strip down aircraft and find damage or failed systems that weren’t part of baseline maintenance but must be remedied to return the aircraft to an airworthy state.
The impact of emergent work is essentially time required to complete work or procure spares, as well as cost of labour, parts and materials.
If an MRO organisation takes a reactive approach to emergent work, it will likely incur higher costs for expedited delivery of parts, and will potentially run into serious schedule issues.
A classical mitigation for this might be to make some provision of risk against cost and schedule - but this isn’t desirable for the MRO or for the customer, and a more proactive approach works better for all involved.
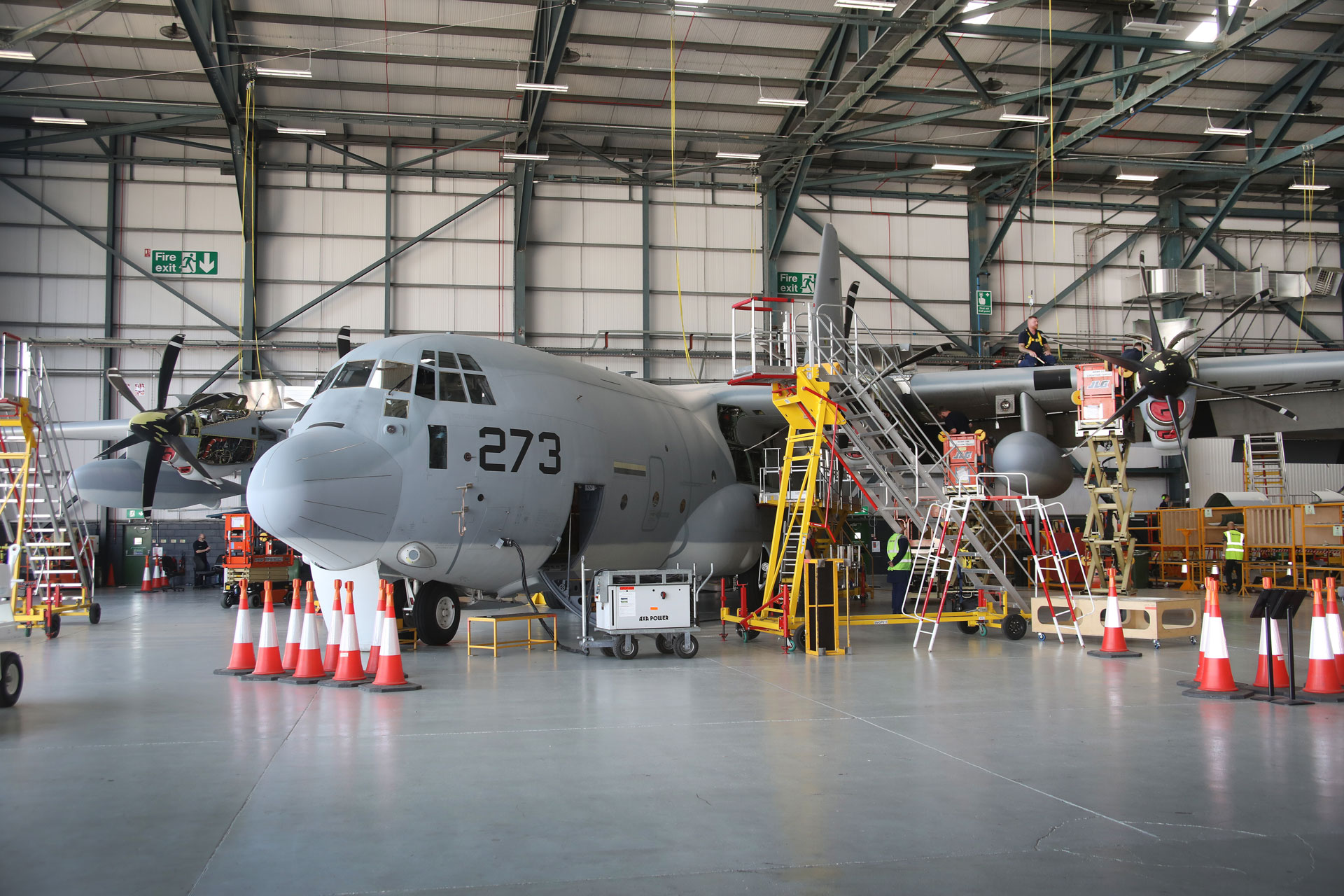
Predicting the needs of an incoming aircraft with a reasonable degree of accuracy, before it arrives, allows an MRO organisation to plan appropriately to direct inspections or procure parts that are likely to be required ahead of time. This will reduce uncertainty and ultimately lead to a better outcome.
An MRO organisation like Marshall will certainly have in-house knowledge and experience that allows some prediction and well informed planning to occur - but such knowledge tends to reside in small teams, and there is limited ability to improve processes by refining existing methods.
We could vastly improve our predictive capabilities - and tailor these to individual aircraft - by using AI to examine the operational and service history of an aircraft, and reference it against datasets containing historical data for similar aircraft (in terms of age, region, maintenance history and operational usage patterns).
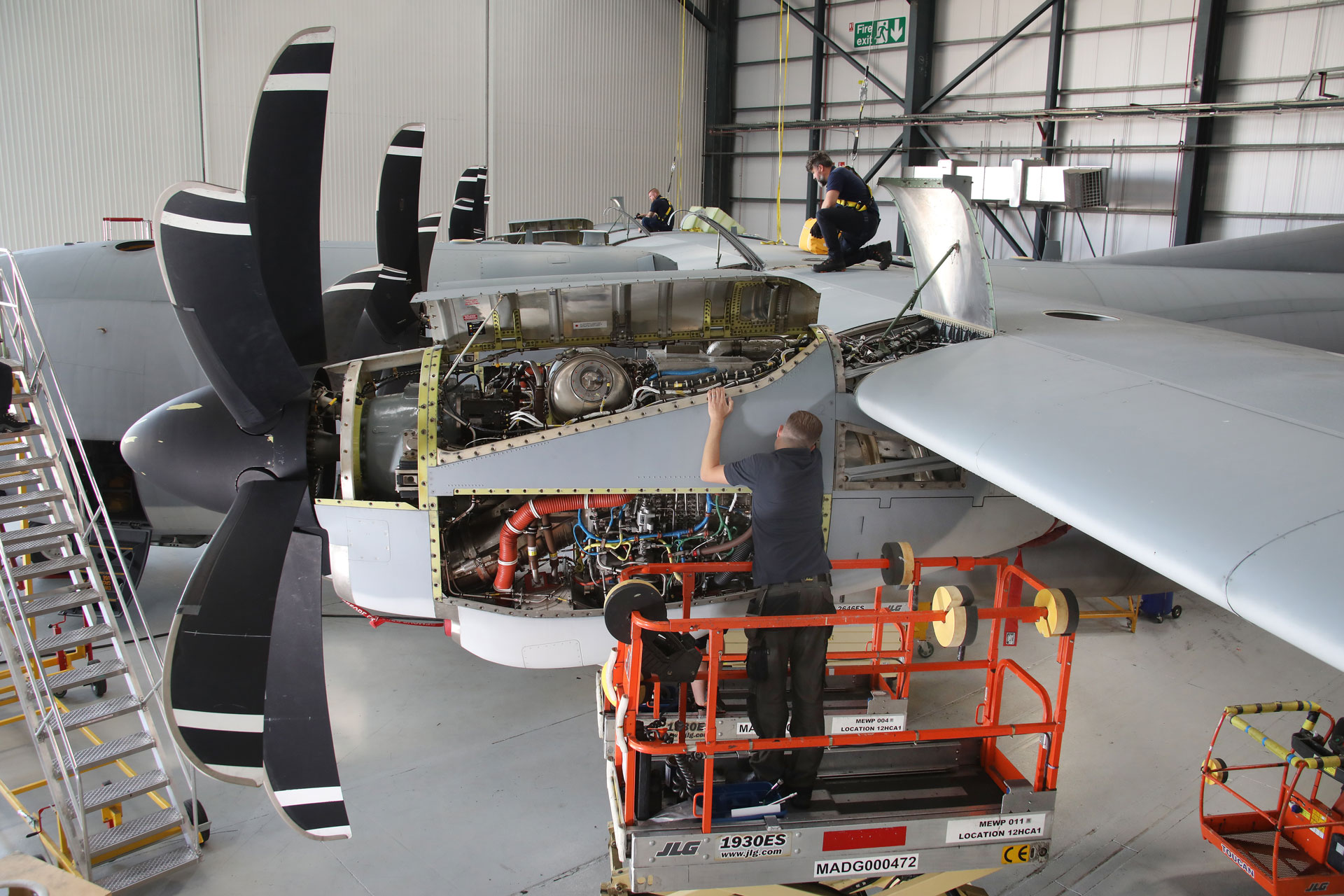
How is it possible to acquire and build the necessary datasets for predictive maintenance?
To a great degree, the datasets we need to work with already exist: for example, the C-130J Super Hercules alone has recorded an incredible 3 million flight hours across over 540 aircraft since the late 1990s - and that’s not to mention the many legacy Hercs in operation worldwide.
At Marshall, we work with 15 global customers on aircraft produced from the 1950s to the 2020s. We can potentially access vast amounts of data - possibly even on par with an aircraft OEM. This includes maintenance and upgrade histories, number of flying hours, operating region(s), and usage patterns. We also have unique data assets in the form of non-routine work cards, which would prove highly valuable as a source of context-rich information on repair and maintenance work.
What AI can do is take that massive amount of data and process it into actionable insights that can be “personalised” to any incoming aircraft. This would enable us to recommend early intervention to prevent deterioration, or perhaps to secure parts with longer lead times.
In saying this, it’s important to recognise that development of any such application and use of the relevant data needs to be with approval from all end users and stakeholders, and in a manner which protects the security of end user data. It’s equally important to recognise a need for transparency with customers where predictions are used in forward planning, and ensure that the limitations of systems of this type are understood.
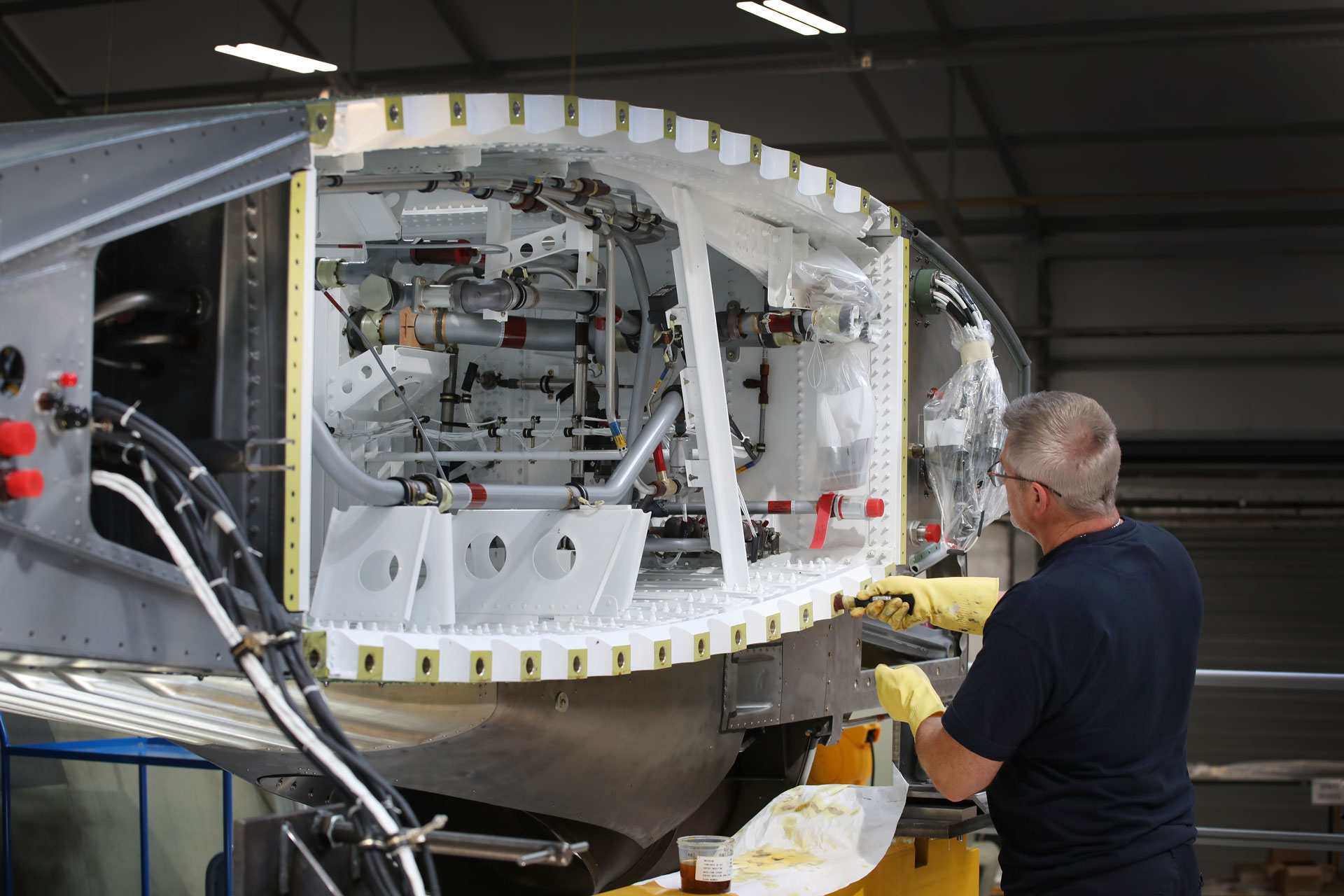
What is task optimisation, and how can AI help?
The objective of task optimisation is to improve efficiency and effectiveness of specific tasks within a process or workflow. Goals might be to increase productivity, reduce tact time, or allocate our resources more effectively. If done well, this could lead to reduced cost and risk, and improved quality.
Most MRO organisations, including ours, will have established Continuous Improvement programmes and operate in a lean, efficient manner. But the work we do is complex, there are many variables, and using data intelligently can help take this further.
We have access to an enormous volume of aircraft history, in addition to millions of hours of documented maintenance work in our hangars. For every task we complete, we gain several data points including time taken, equipment used, technician skill codes, and outcome. These can then be associated with the broader contextual story around the work performed on that individual aircraft.
Spotting patterns in such a large and varied dataset is often beyond the capability of humans and more traditional methods. AI really performs in this space, and a combination of unsupervised and supervised learning techniques can help us to either predict the outcome of a complex series of events, or spot correlations we didn’t know existed.
So the outputs of AI might help us to derive significant actionable insights that might get overlooked by more traditional methods.
This kind of meaningful causal insight would prove extremely valuable in helping MRO organisations shave hours of the time they need to spend working on each aircraft, and would also likely inform the development of new best practices.
Who benefits most from this? The MRO organisation or its customers?
The short answer is: both.
AI-powered task optimisation could help MRO organisations work more efficiently and effectively, increasing their capacity and flexibility around scheduling work while maintaining the same facilities and resources.
If you’re a fleet operator, you would feel the benefit in terms of turnaround times: your aircraft would be returned to service sooner, thereby boosting overall fleet availability. Ultimately, that’s something all our customers are seeking!
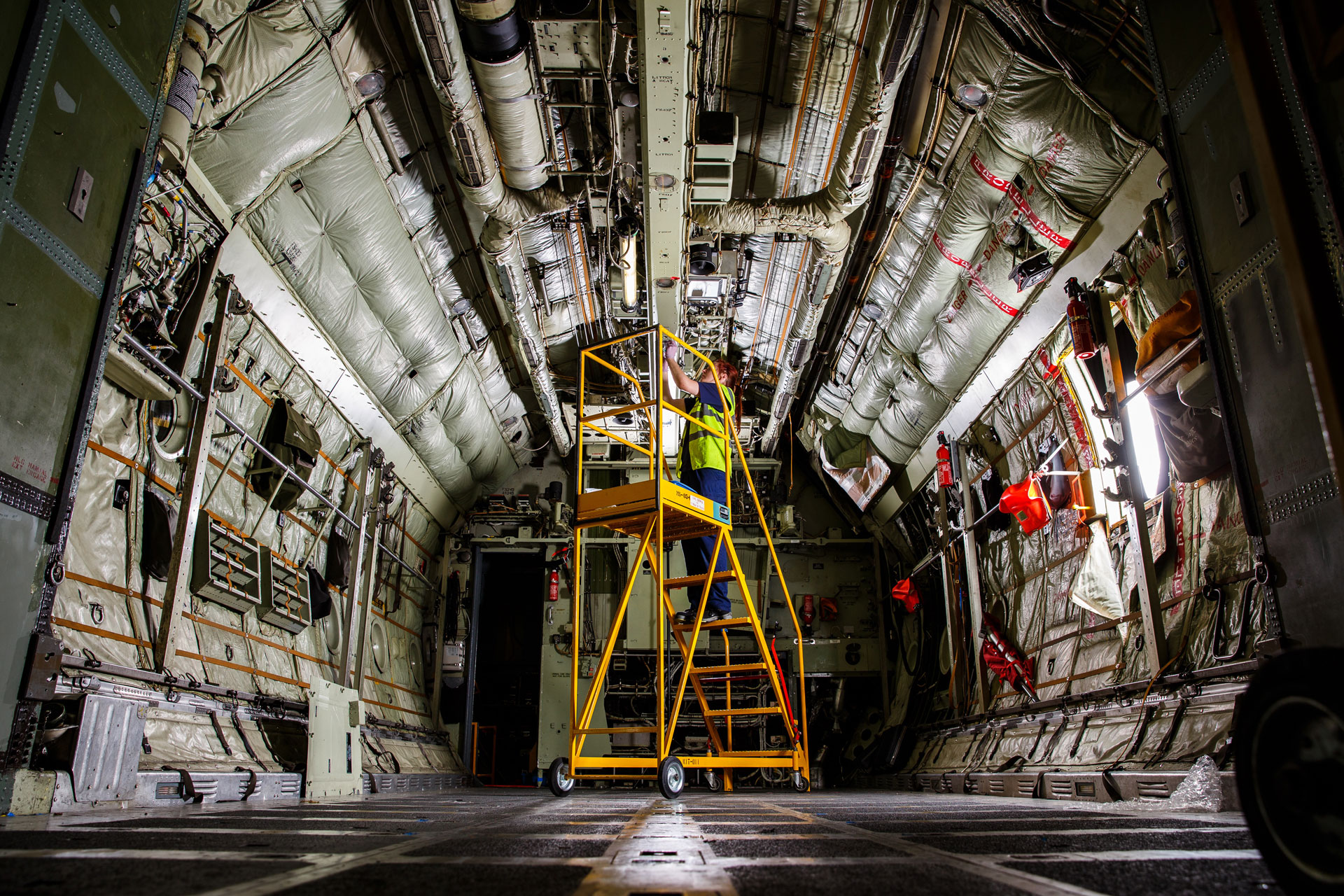
What would be some examples of using AI to augment human practice?
This is a big topic so we should perhaps start by saying that the objective of augmented human practice is to enhance human capability and create scenarios where people and supporting technologies can achieve something together which they might not be able to do alone. So this might be using technology to do something better, to learn something new or to aid decision making.
So in an MRO context, one example might be in general visual inspection of an aircraft. In this scenario a technician will spend a large amount of time completing inspections and then documenting what they’ve found and determining action to be taken.
If you add AI technology into this scenario, computer vision combined with a database of images might very quickly establish where damage exists and direct a technician to focus on these areas first, or highlight areas of concern. The same system then might assist in the meticulous digital cataloguing of damage and generation recommended actions.
Another example might be in coaching or training a technician to complete infrequent or complex tasks.
AI can support technicians in these situations by combining instant access to relevant manuals and training resources from within our systems, or by observing a task and providing coaching and feedback.
If done well then these systems should, over time, help to develop the skills and competencies of a team, rather than creating a dependency on those systems. A lot of research is being done in this area.
Do you see AI replacing the work of human MRO technicians?
Put simply, no. As humans we are uniquely capable, versatile and adaptable. We also can also learn and gain insight from much less data. There will always be scenarios where human knowledge and human ability is critical.
So it’s important to recognise the current limits of technology. In a safety-critical context, tools like AI should be used to enhance human decision making and as an aid to efficiency. Decision-making and quality assurance of outputs will remain a human activity, and human accountability should always exist.
But alongside recognising the limitations, we should also recognise the benefits. I don’t know anybody that got into engineering for the paperwork, or to spend hours upon hours reading documents. AI can help automate and improve efficiency in these areas, allowing more time to be spent in the value-adding and rewarding parts of our jobs - including training and coaching others.
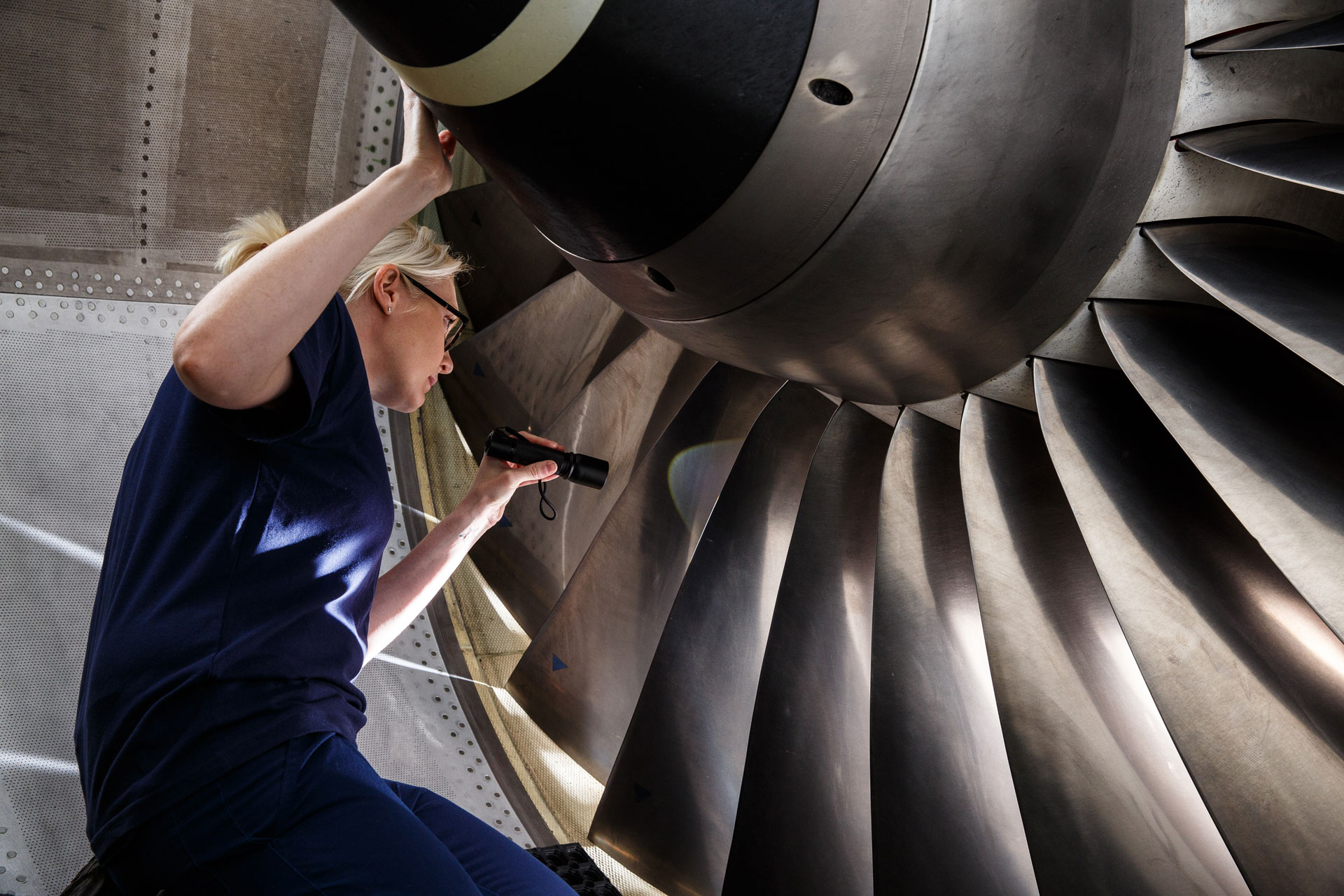
What do you see as the biggest barriers to adoption for AI in MRO?
The first and most obvious obstacle is the fact that we are all working in a very tightly regulated space - in fact, this is a topic that probably merits an entire series of articles in its own right! Regulations exist for good reason, and thankfully there are a lot of informed and open-minded discussions between regulatory bodies and experts regarding the best approach to gradually introduce AI-driven technologies and approaches in a safe and responsible manner. So, while we certainly won’t see sudden and transformative changes, I would expect steady progress over the next few years.
Looking beyond regulatory and privacy issues, data quality is fundamental to success. This is true throughout the AI and machine learning landscape. The quality of predictions and recommendations made by a model will always depend heavily on the quality of training data used, and appropriate selection of an algorithm or design of a neural network. This kind of “rubbish in, rubbish out” problem can be largely avoided by ensuring data points represent all of the variation which may exist in a scenario and are free from any bias.
But alongside this, aircraft are, by design, incredibly reliable with very low failure rates. AI is very data-hungry, so whilst an organisation might hold a vast number of records, there might only be a small number of instances of a particular failure or outcome we would be interesting in predicting. So we need to be very intelligent about how we use that data to gain meaningful insight, or how we supplement data using complimentary technologies such as high fidelity simulation.
Lastly, I would say that MRO organisations looking to implement AI need to ensure that predictions and recommendations are, in fact, actionable. Useful and actionable are not the same thing: a suggested course of action could have great potential in an ideal world, but it might also be completely incompatible with the required operating processes and practicalities that are specific to MRO - rendering it useless. The challenge for MRO organisations will be designing models in a way that ensures they provide predictions and recommendations in line with these constraints.
What considerations should be top of mind for MRO organisations taking their first steps in AI?
For an organisation like Marshall, AI should never undermine a well-earned reputation for technical excellence and world-class solutions that perform safely and reliably.
This means that any AI solutions we develop and deploy must reflect these values by following appropriate industry-leading development practices, and undergoing thorough testing and evaluation to ensure the quality and validity of outputs.
Even after deployment, the technical robustness of any solution should be maintained throughout its operational life.
Just as importantly, AI solutions need to be implemented in an ethically sound manner, with respect for customers. Privacy of data should be protected by design, communication around model usage must be transparent, and customers must be represented equally and fairly.
If you’re starting out on your journey in AI, then I recommend spending some time considering the core principles that would guide any implementation. These might include how you ensure applications are safe, technically robust, respectful of customer privacy and security, and that you have the right systems and processes in places to ensure human accountability and responsible use.
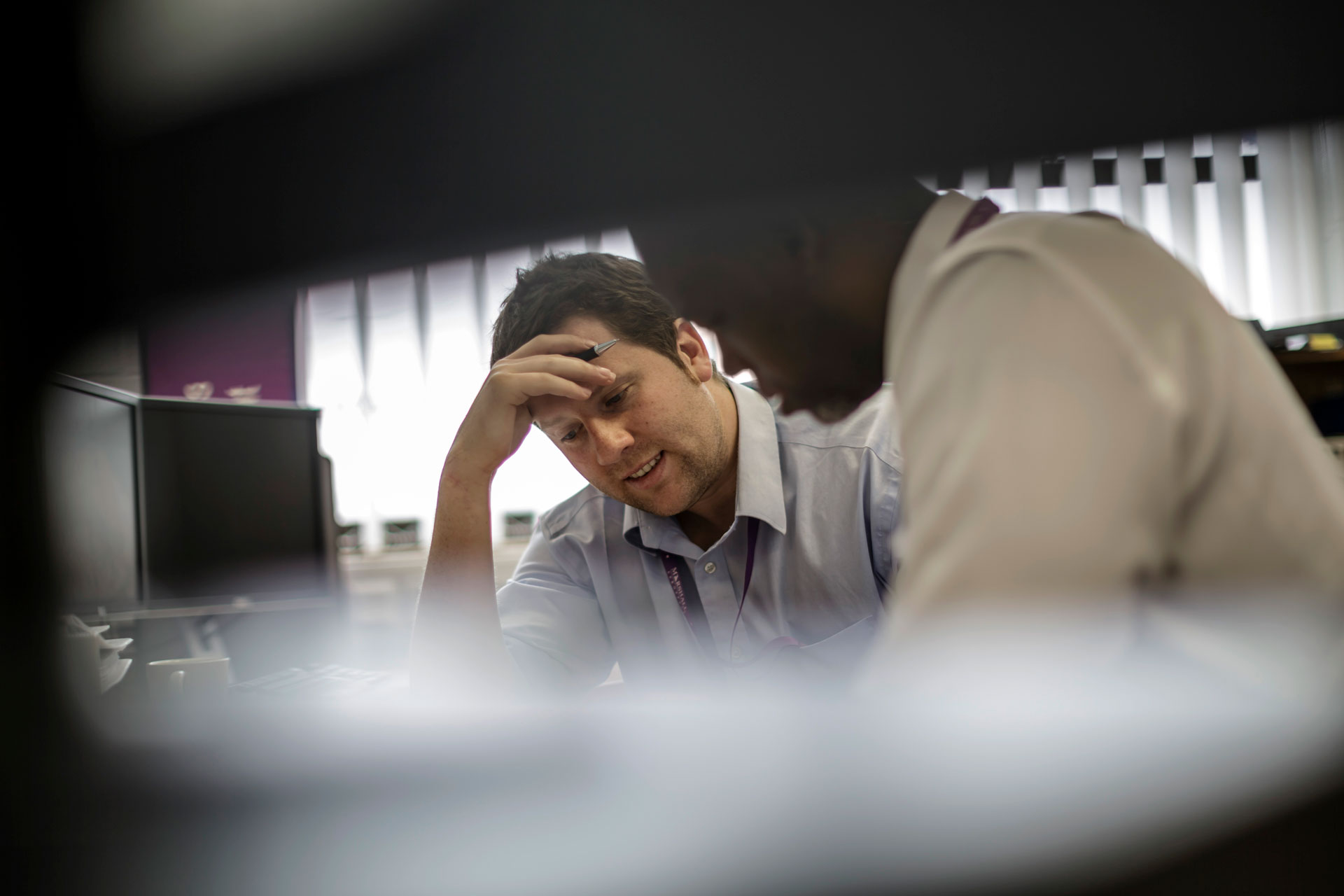
It’s also worth spending some time considering what your unique data asset is. Lots of off-the-shelf tools and applications exist, and these will become common in most businesses. So it’s important to understand what unique data you hold that might give you an advantage.
Lastly, AI implementations are most successful and valuable when they work for the individual, the business, and are sensitive to the wider context of job displacement and disruption.
Note: the image at the top of this page was created using Microsoft Copilot, powered by DALL-E 3.